Artificial Intelligence (AI) is often described as smart, efficient and superhuman. That sounds quite nice, but it leaves out a lot of details. In her book Atlas of AI, Kate Crawford maps out the power systems, impact on society and stakes that come with deploying AI. That’s important, because we, and the systems that affect us, increasingly rely on these technologies.
There are lots of worthwile pursuits in AI: we can make computers understand our speech, teach medical devices to recognise irregularities in scans and analyse financial transactions for fraud. But there is plenty of applications that may not actually contribute all that much, or are downward hostile if you consider what could go wrong. Systems deployed to surveil workers, filter resumes or drive cars (sorry) may not be worth their damage.
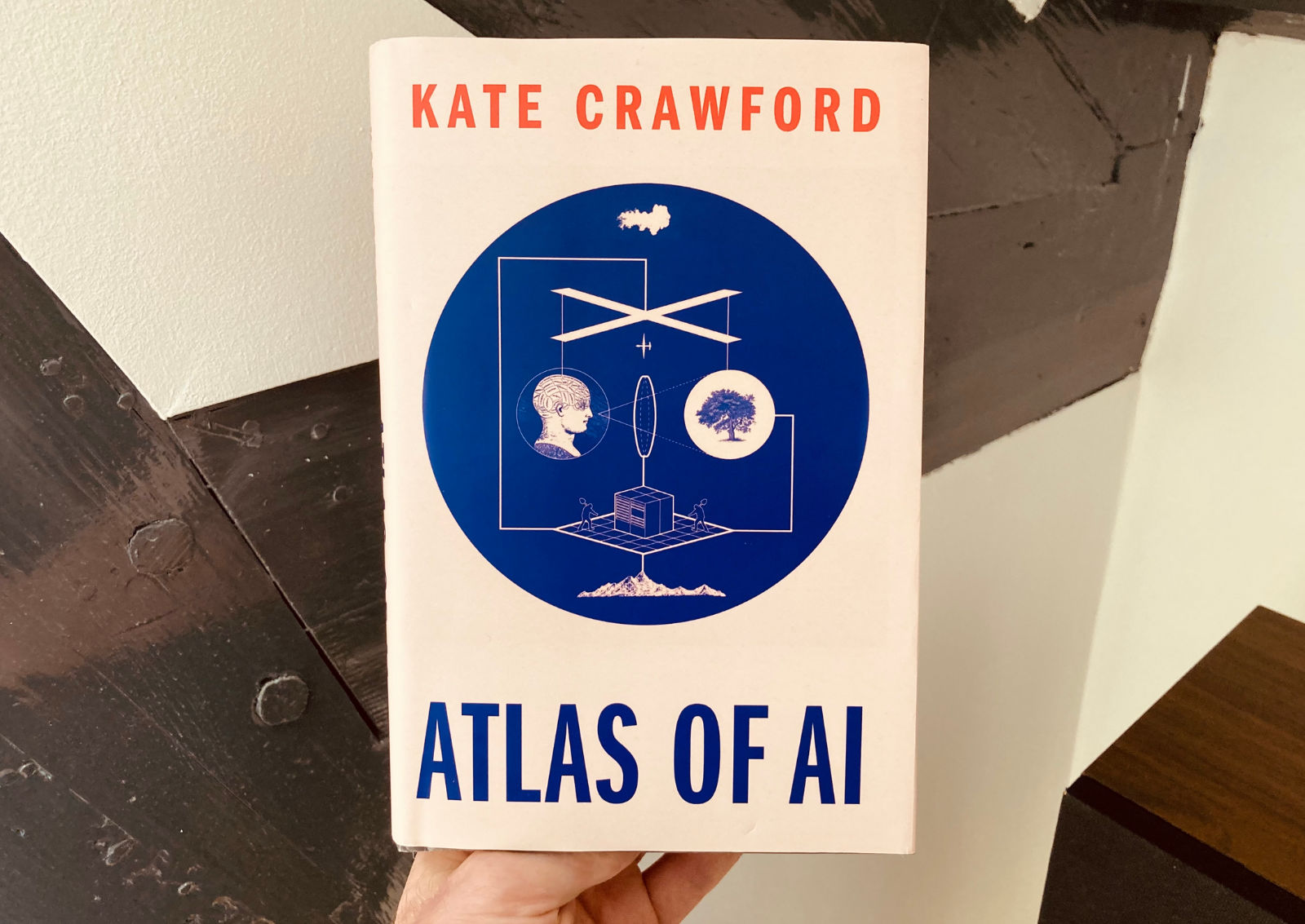
Natural resources and people#heading-1
The impact of AI starts with the raw materials of machines, Crawford explains. Computers need chips and those require rare earth materials like tin. It’s easy to forget about that: mining such materials isn’t without consequences. It badly affects workers (and their conditions when working in mines), their direct environment (pollution) and the earth at large. Once the chips exist and machines run, there is also an environmental impact. The data centres that compute our data require enormous amounts of gas, water and electricity. One of the largest US data centres uses 1.7 million gallons of water per day, is just one of the many examples Crawford provides. When a company says ‘our product uses AI’, their product also uses these raw materials, these data centres and this energy.
Reassuringly, most big tech companies have very green ambitions, like powering 100% of their data centres with renewable energy. This is good. But when not all of the world’s energy is renewable, we’ve got to be sure there are good reasons for any energy that is used. We’ve got to focus on using less of it, and endlessly processing ginormous amounts of data does not help.
Input data and classification#heading-2
Crawford also writes about the basic ingredient for AI and specifically machine learning: data. To teach machines about a thing, we need to show them examples of the thing. To produce AI, a company needs to collect data, ‘mine’ it, if you will. Data, like text messages, photos, audio or video. It is often collected without the consent of the people involved, like the people who are in the photos. Crawford warns us against ‘the unswerving belief that everything is data and is there for taking’ (AAI, 93).
There are exceptions, like Common Voice, a Mozilla project that aims to ‘teach machines how real people speak’ by asking people to ‘donate their voice’. The teaching machines part is not unlike other machine learning projects, but the data collection is. Common Voice data is not taken from some place, people provide it consentually and for the very purpose of training machines. This is uncommon (pardon the pun).
And then there is the issue of context. When images become data, context is considered irrelevant, it no longer matters. They serve to optimise technical performance and nothing else. This data is now seen as neutral, but, explains Crawford, it is not:
‘Even the largest trove of data cannot escape the fundamental slippages that occur when an infinitely complex world is simplified and sliced into categories’ (AAI, 98)
Taking data out of context also doesn’t remove all context. A data set could affect privacy in unexpected ways. Crawford discusses a set of data from taxi rides, that may have looked quite innocent and useful from the outset. What could go wrong? Well, from the data, researchers could deduce religions, home addresses and strip club visits.
Classification
Machine learning requires input data to be classified. This is a fire hydrant, that is a bicycle. Some firms pay people through Mechanical Turk… there are workers who spend all day describing images. Other companies demand the work to be done for free by users who want to login (hi recaptcha).
Perhaps more important than who does the work are the philosophical problems of classification. Is there a fixed set of categories? Does everything neatly fit in them? Such questions have boggled philosophers’ minds for, literally, millenia. It’s safe to say most would say no to either question. Yet, AI firms seem more confident. They have to, as classification of data is at the heart of how machine learning works.
The harm caused by classification problems depends on the subject, but it is widespread. Maybe the AI that was trained with some wrongly classified fire hydrants won’t cause too much trouble. But there are endlesss examples of AI exhibiting awful bias, including towards race and sexual orientation. Bias in AI has been seen as a bug to be fixed, but it should be seen as a problem of classification itself. ‘Classification is an act of power’, says Crawford (AAI, 127). The categories can be forgotten and invisible once their work in the model training phase is over, but their impact remains.
You can’t fix bias by merely diversifying a set of data, Crawford says:
‘The practice of classification is centralizing power: the power to decide which differences make a difference’ (AAI, 127)
Sometimes researchers measure the wrong thing, because are constrained by what they can measure. For example, it’s easy to measure skin colour, but it’s not the thing to measure if you’re after understanding something about race or ethnicity. It doesn’t work that way… ‘the affordances of the tools become the horizon of truth’, Crawford concludes.
Open data collections often show categories that reinforce racism and sexism, and that’s just the sets we can see. Is there a reason to assume the sets of Facebook and Google avoided these problem?
Emotion recognition and warfare#heading-3
The last two chapters of “Atlas of AI” consider two of the scariest answers to ‘what could possibly go wrong?’: AI tech for emotion recognition and warfare.
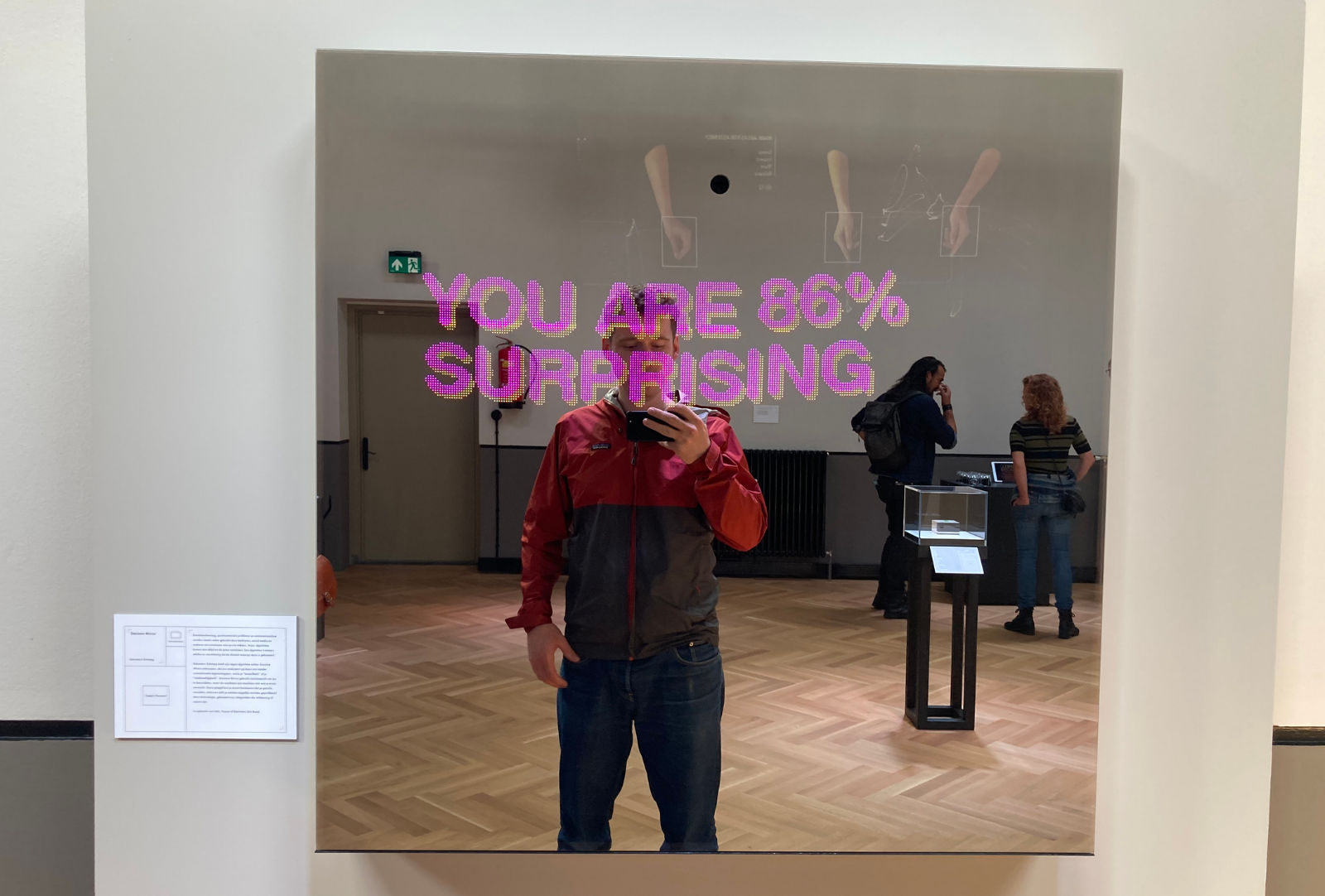
Me at The Glass Room in Leeuwarden in front of an art work about emotion recognition
Research in emotion recognition, Crawford writes, comes from a ‘desire to extract more information about people than they are willing to give’ (AAI, 153). It is already in use by HR departments to scan applicants and try and match their facial expressions to personality traits.
Can it work? Scientists including Paul Ekman tried to find out whether emotions are universal. Some were confident, but as of yet there is no consensus on which emotions exist and how they manifest (AAI, 172). Crawford explains there is growing critique doubting the very possibility that there is a clear enough relationship between facial expressions and emotional states (AAI, 174). It’s merely correlations, she says:
In many cases, emotion detection systems do not do what they claim. Rather than directly measuring people’s interior mental states, they merely statistically optimize correlations of certain physical characteristics among facial images (AAI, 177)
So, like with other AI systems, AI for emotion recognition relies on categorisation, which Crawford explained is flawed and an act of power, so it has many of the same problems:
[An analysis of the state of emotion recognition] returns us to the same problem we have seen repeated: the desire to oversimplify what is stubbornly complex, so that it can be easily computed, and packaged for the market.
And while this may work for companies selling AI systems, the end result is likely a lack of nuance:
AI systems are seeking to extract the mutable, private, divergent experiences of our corporeal selves, but the result is a cartoon sketch that cannot capture the nuances of emotional experience in the world.
Governments use AI for warfare, in the US the ‘Third Offset’ strategy includes leveraging Big Tech to create infrastructure of warfare (189). In “Project Maven”, the US Department of Defense paid Big Tech firms to analyse military data from outside the US, like drone footage, to build AI systems that could recognise ‘vehicles, buildings and humans‘ (AAI, 190)
Crawford describes a shift from debating whether to use AI in warfare at all, to, as former Google CEO Eric Schmidts said, whether AI would be able to ‘kill people correctly’ (AAI, 192)
‘Militarised forms of pattern detection’, Crawford explains, go beyond national interests when companies like Palantir sell their technology widely (eg local law enforcement and supermarket chains). They are focused not on enemies of the state, but ‘directed against civilians’. Machine learning based patten recognition tech is used to find ‘illegal’ immigrants to deport (‘illegal’, because no humans are illegal). In Europe, IBM was tasked to use data analysis to assign refugees a ‘terrorist score’, capturing the likelihood of them being a terrorist. This would be a bad idea if AI systems were 100% able to make such distinctions, and let’s be clear: they can never be. Because of the inevitability of bias and the very nature of categorisation. If even humans don’t universally agree about what constitutes a terorrist, and they don’t, how can any human categorisation we do successfully help teach machines?
When systems impact government decisions, they requires oversight, and there is very little of that, explains Crawford (AAI, 197). Without that, we risk making things worse, she says:
Inequity is not only deepened, but tech-washed, justified by the systems that appear immune to error yet are, in fact, intensifying the problems of overpolicing and racially biased surveillance
Summing up#heading-4
Atlas of AI provides insight in what we give up when employing AI. There may be applications that are mostly helpful and little harmful, like language. There are applications that probably harm more than help, like emotion and warfare.
The book also reassures us that some things may not be as computable as they seem. Good categorisation is hard for humans and harder for machines. There is this fantastic tv show broadcasted on Dutch television called Zomergasten: a person of interest is interviewed for 3 hours straight on live television, and they bring in their favourite video fragments. YouTube may have state of the art machine learning powered recommendation engines, the suggestions these people bring often delight and surprise me more.
Above all, Atlas of AI will give you a fresh perspective that you can use when you read about the next thing AI is claimed to solve. Yes, the possibilities are promising. The features often cool. But we’ve got to keep our feet on the ground and assess AI technologies carefully.
Comments, likes & shares (13)
Tristan Ferne, breaque, Miriam (still), DCoder ????????❤????????, JP, Joe Gaffey, lj·rk and Jean Hominal liked this
Baldur Bjarnason, Fran, Sietske Boer-van Vugt, lj·rk and Jean Hominal reposted this